A prognostic machine learning model for the prediction of pancreatic adenocarcinoma prognosis based on genomic expression of four cell-cycle associated hub genes
Highlight box
Key findings:
• Four genes of cell cycle (TPX2, KIF20A, CCNB2, and NCAPH) were found to affect the prognosis of pancreatic adenocarcinoma (PAAD) patients poorly when overexpressed.
What is known and what is new?
• Higher levels of expression of certain hub genes can affect prognosis of pancreatic cancer and other types of cancer either by increasing or decreasing their survival rates. For example, MMP7 and ITGA2 were identified as hub genes that affect pancreatic cancer prognosis and diagnosis.
• Upon using machine learning technique characterized by high accuracy, we discovered that four hub genes regulating normal cell-cycle were over-expressed in pancreatic adenocarcinoma patients and associated with poor prognosis and overall survival.
What is the implication, and what should change now?
• Identification of these hub genes can greatly improve prognosis and survival of pancreatic adenocarcinoma patients by targeting them for the development of anti-mitotic therapy.
Introduction
Pancreatic adenocarcinoma (PAAD) is one the most aggressive tumors ranking the seventh and third cause of cancer-related deaths worldwide and in the United States respectively. It arises from the epithelium of the pancreatic duct, accounting for more than 90% of pancreatic cancer diagnoses. Patients with PAAD present with a poor prognosis and 5-year survival rates are less than 5% (1). The diagnosis of PAAD remains a devastating process, as patients are often diagnosed at late stages with late presenting symptoms which contribute to high-lethality and aggressiveness of the tumor. The presentation of symptoms depends on the tumor’s location, but pain, weight loss, and jaundice are the most common symptoms (2).
The cornerstone of advanced PAAD treatment is cytotoxic chemotherapy, mainly gemcitabine monotherapy as a first-line therapy, or doublet and triplet combinations with other agents (3,4). However, this modality results in poor overall survival (OS) of less than 1 year (5,6). PAAD is characterized by an immunosuppressive environment, causing high resistance to treatment and fatality rate (7). Thus, studies are now directed to investigate the efficacy of drugs targeting genomic alterations in PAAD. Immunotherapy efficacy has shown controversial results in patients with PAAD, as the use of anti-programmed cell death protein-1 (anti-PD-1)/anti-programmed death-ligand 1 (anti-PD-L1) and anti-cytotoxic T lymphocyte-associated protein 4 (anti-CTLA4) did not reveal any activity of immune checkpoint inhibitors in advanced PAAD but showed efficiency in PD-1 blockade in PAAD patients with mismatch repair deficiency (8).
Cell cycle control is one of the most substantial processes to maintain normal tissue growth, and abnormal regulation of this process may promote cancer proliferation or inhibit apoptosis. Many genes have shown a regulatory function in cell cycle phases, however, overexpression of some genes was associated with increased proliferation and evasion of apoptosis (9). In PAAD, deregulation of cell cycle kinases has shown to be associated with its tumorigenicity, thus, genes associated with kinase pathway and show upregulation in PAAD can be used as a therapeutic target for the development of cell cycle kinase inhibitors (10).
As the early diagnosis of PAAD presents a core hardship that affects patients’ prognosis, the introduction of machine learning (ML)-based models has been proposed to facilitate PAAD diagnosis and increase patients’ prognosis. ML algorithms can be used to classify cancer patients into high and low-risk groups, which allows the study of the clinical significance across groups (11). These models work by encompassing several variables including gene expression profiles, histological parameters, and clinical variables in a complementary manner to act as training parameters to predict patients’ prognosis (12). The most common types of ML models used in cancer detection are artificial neural networks and decision trees (13,14), these models allow researchers to combine data from multiple sources and use them for prognosis prediction or tumor detection (15). To rate the accuracy of these models, several validation methods can be used, including the training-validation method, which splits the dataset into a training set to identify the molecular signature and a test set to rate the amount of misclassifications (16).
The implementation of ML models on gene expression profiles by the use of DNA microarrays can help predict patients’ clinical outcomes such as prognosis or tumor staging. Web-based databases for cancer genomics such as The Cancer Genome Atlas (TCGA) project represent an important advance to improve the knowledge and understanding of cancer and related genes, providing a large number of patients’ data to integrate them into bioinformatic analyses such as gene expression patients’ survival (17).
In this study, we aim to integrate the genomic expression of cell-cycle associated genes to build and evaluate an ML model to predict the prognosis in PAAD, and to investigate the expression of these genes in PAAD compared to normal tissue and the relation between their levels of expression with patients’ survival.
Methods
Data acquisition
A thorough search through genomic and transcriptomic databases was conducted using TCGA (https://www.cancer.gov/tcga) and cBioportal databases. The TCGA is a genomic program that contains over 20,000 primary cancer molecular, genomic, transcriptomic, and proteomic data on 33 cancer types with matched normal samples. This database was used to obtain clinical and expression data of PAAD patients from the Pan-Can project. The cBioportal is an open-source cancer genomic database developed at Memorial Sloan Kettering Cancer Center (MSK) (18,19). It consists of cancer genomic and clinical data from different projects, including the TCGA project. In this study, we selected and downloaded the data from cBioportal based on the following criteria: (I) samples of PAAD; (II) samples reporting the mRNA expression as z-scores relative to all samples; and (III) samples for the corresponding mRNA expression with data on OS status in months. Studies that reported mRNA expression without OS data or reported OS data without mRNA expression were excluded.
Gene Expression Profiling Interactive Analysis 2 (GEPIA2)
The GEPIA2 is a webserver for transcriptomic analysis that provides an interactive framework to analyze RNA sequencing expression of tumor samples from the TCGA project and normal tissue samples from the Genotype-Tissue Expression (GTEx) project (20). This database was used to carry out the significantly survival-associated gene analysis and validate their expression using the differential expressed genes (DEGs) tool in PAAD by analyzing the differences in the abundance of gene transcripts within mRNA sequence (transcriptome), which aid in the identification of potential biomarkers for multiple cancers and targeted therapies. The DEGs were analyzed using a |log2FC| cutoff value of 2 and q-value cutoff of 0.05 for PAAD tissue matched with normal tissue samples from TCGA and GTEx databases.
Protein-protein interaction (PPI)
The resulting survival-associated genes were analyzed using the Search Tool for the Retrieval of Interacting Genes/Proteins (STRING) database, which examines the significantly enriched genes for biological processes, cellular component, molecular function, and Kyoto Encyclopedia of Genes and Genomes (KEEG) pathways (21). PPI network was constructed for the resulting survival-associated genes from the GEPIA2 database for confidence network and a minimum interaction score of 0.90 representing the highest confidence and 1% high false discovery rate (FDR).
Survival analysis using Kaplan-Meier plotter
The Kaplan-Meier plotter is an online tool to assess the correlation between mRNA, miRNA, DNA, or protein expression of over 30,000 genes and the survival data of over 25,000 samples from 21 cancer types from different databases including the TCGA project, thus providing survival biomarkers for the cancer and gene of interest (22).We used this tool to assess the correlation between mRNA sequence expression of the previously chosen genes from GEPIA2, and the OS data of PAAD patients from the TCGA Pan-Can project, by splitting the mRNA expression samples of our proposed markers into two groups of low levels of expression and high levels of expression, then the two groups are compared using Kaplan-Meier survival plot and a hazard ratio (HR) with 95% confidence intervals and log-rank P values.
ML classification ensemble
To provide a diagnostic model for the chosen genetic markers, we built an ML classification ensemble, to predict the prognosis of PAAD and survival status. The obtained mRNA expression data of PAAD patients from the cBioportal database were used to construct the model, mRNA expression z-scores were set as feature variables, and the OS status was denoted as the target variable. The sample was randomly split into 80% training set and 20% testing set. Figure 1 illustrates data processing for the ML model.
The training set was fitted into a Random Forest Classifier (RFC) ensemble. The model’s performance was then evaluated using the mean bootstrap estimate with 95% confidence interval (CI). To avoid overfitting, we used a 10-fold cross-validation method, in which the model is trained using k−1 fold as the training data, and then the model is validated on the rest fold of the data. A cross-validation methodological illustration is shown in Figure 2. A cox proportional hazard (CPH) model was adjusted for the expression levels of the obtained genes in a univariate and multivariate fashions. The RFC model was built and evaluated using Scikit-learn (v1.0.2) package from Python (v3.9.7), and the CPH model was built using survival and finalfit packages from R language (23).
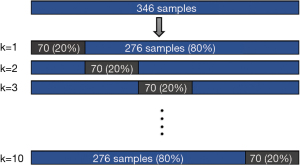
Ethical clearance
The study conformed to the provisions of the Declaration of Helsinki (as revised in 2013). This study did not require ethical approval. All the data used in this study were obtained from open databases (cBioportal) of de-identified individuals.
Results
Sample characteristics
The cBioportal database identified 16 datasets on pancreatic cancers, with 3,592 patients (1,901 males, mean age =65.57 years) with PAAD. A total of 346 samples (314 males, mean age =64.71 years) from two datasets reported z-scores of mRNA expression for the proposed genes with their corresponding OS data and were entered for further analysis. Treatment modalities for these patients included 102 patients on gemcitabine, 45 on radiation therapy and 51 on other chemotherapeutic agents.
Candidate gene selection
A total of 500 significant survival-associated genes in PAAD were identified from the GEPIA2 database, which were further analyzed for PPI interaction using the STRING database. The PPI network analysis resulted in 19 clustered genes, which were further analyzed for differential expression in PAAD and normal tissue. After the differential expression analysis, we identified a total of 4 genes that were both significantly upregulated and associated with PAAD survival. These genes included: TPX2, KIF20A, CCNB2, and NCAPH. All these genes showed significant overexpression in PAAD compared to normal tissue: TPX2 [log2(TPM +1) median: 3.4 vs. 0.12], KIF20A [log2(TPM +1) median: 2.34 vs. 0.1], CCNB2 [log2(TPM +1) median: 3.3 vs. 0.3], and NCAPH [log2(TPM +1) median: 2.32 vs. 0.14]. Boxplots for genes’ expression between PAAD and normal tissue are shown in Figure 3.
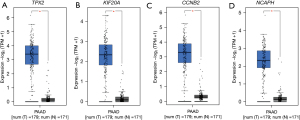
Gene expression and disease progression
The survival analysis of all genes showed better prognosis and higher OS in patients with low levels of gene expression: TPX2 [OS (HR) =3.24; 95% CI: 1.56–3.6; log rank P value <0.000001], KIF20A [OS (HR) =2.23, 95% CI: 1.45–3.43; log rank P value <0.0001], CCNB2 [OS (HR) =2.54, 95% CI: 1.64–3.94: log rank P value <0.0001], and NCAPH [OS (HR) =2.34; 95% CI: 1.5–3.63; log rank P value <0.0001]. Kaplan-Meier plots for the survival of each gene are shown in Figure 4.
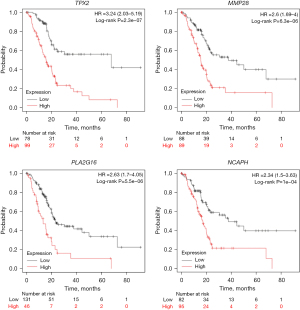
Prognostic ML classification model
The training set of each model comprised 276 patients (80%), and the test/validation set comprised 70 patients (20%). The classification model for the TPX2 gene predicted OS with a mean accuracy estimate of 83% and 95% CI: 0.75–0.89, and a mean 10-fold cross-validation score of 0.51. Whereas for the KIF20A gene, the model performed with a mean accuracy of 68% and 95% CI: 0.62–0.74, and a mean 10-fold cross-validation score of 0.41. For the CCNB2 gene, the model was able to predict OS with a mean accuracy of 79%, 95% CI: 0.75–0.85, and a mean 10-fold cross-validation score of 0.56. While the NCAPH classification model predicted the OS with a mean accuracy of 81%, 95% CI: 0.76–0.87, and a mean 10-fold cross-validation score of 0.56. All models’ evaluation scores are presented in Table 1. The concordance index (C-index) of the CPH model was 0.64, logrank test P value <0.0001. The HR for the univariate analysis showed increased risk and worse prognosis (P value <0.001), when adjusting for the multivariate analysis, KIF20A and TPX2 genes showed increased hazard risk (P value <0.05), while the NCAPH gene showed decreased hazard risk (P value <0.05). The CCNB2 gene did not show significant prediction in the multivariate analysis. Table 2 shows the HR and P values for the univariate and multivariate analysis.
Table 1
Model | Mean bootstrap estimate | 95% CI | 10-fold cross validation |
---|---|---|---|
TPX2 | 0.83 | 0.75–0.89 | 0.51 |
KIF20A | 0.68 | 0.62–0.74 | 0.41 |
CCNB2 | 0.79 | 0.75–0.85 | 0.56 |
NCAPH | 0.81 | 0.76–0.87 | 0.56 |
Combined | 0.86 | 0.79–0.92 | 0.58 |
CI, confidence interval; TPX2, targeting protein for Xenopus kinesin-like protein 2; KIF20A, kinesin family member 20A; CCNB2, cyclin B2; NCAPH, non-structural maintenance of chromosome condensin I complex subunit H.
Table 2
Variables | Mean (SD) | Univariate | Multivariate | |||
---|---|---|---|---|---|---|
HR (95% CI) | P value | HR (95% CI) | P value | |||
KIF20A | 0.1 (0.9) | 1.89 (1.50–2.39) | <0.001* | 1.68 (1.13–2.51) | 0.010* | |
TPX2 | 0.0 (0.9) | 1.77 (1.45–2.16) | <0.001* | 1.02 (0.65–1.59) | 0.012* | |
NCAPH | 0.1 (0.9) | 1.56 (1.29–1.87) | <0.001* | 0.63 (0.40–0.98) | 0.039* | |
CCNB2 | 0.1 (0.9) | 1.66 (1.36–2.02) | <0.001* | 1.86 (1.15–3.02) | 0.938 |
*, P value <0.05. SD, standard deviation; HR, hazard ratio; CI, confidence interval; TPX2, targeting protein for Xenopus kinesin-like protein 2; KIF20A, kinesin family member 20A; CCNB2, cyclin B2; NCAPH, non-structural maintenance of chromosome condensin I complex subunit H.
Discussion
PAAD is the most common type of pancreatic cancer accounting for 90% of the cases and is associated with a very poor prognosis and less than 9% 5-year survival. Patients with PAAD are diagnosed in very late stages, presenting with advanced and metastatic transformation to other sites (24). Therefore, in this study we aimed to identify survival-related genetic biomarkers that are significantly associated with PAAD progression and showed upregulation in pancreatic tissue compared to normal tissue. We also provided an ML-based prognostic tool, which predicts the OS based on the mRNA sequence expression of the identified genetic biomarkers, hence, improving PAAD prognosis and diagnosis.
The TPX2 gene is a microtubule-associated gene that plays an important role in mitotic spindle formation and chromosomal segregation (25). The PPI network analysis showed significant enrichment of this gene in mitotic biological processes and a kinase molecular function. Differential gene expression analysis revealed that it was significantly upregulated in PAAD, which is associated with high pancreatic tissue proliferation. This gene has been shown to activate the Aurora A kinase activity by arresting it in the active form (26). High levels of expression of this gene were significantly associated with a poorer prognosis in PAAD. A cell line study by Warner et al. (27) revealed that the knockdown of this gene was associated with increased apoptosis in pancreatic tissue, and the effect of its inhibition on the cytotoxicity of taxanes in pancreatic tissue resulted in mitotic arrest and decreased cell viability. Thus, these results indicate that this gene can be used as a target for the development of anti-mitotic drugs for PAAD.
Another genetic biomarker that has been associated with mitotic cellular processes is the KIF20A gene from the kinesin family. This gene showed significant overexpression in PAAD compared to normal tissue, and it was also associated with patients’ prognosis. It has also been shown to act as a carcinogen associated with poor prognosis in other cancer types, including medulloblastoma (28), gastric cancer (29), and hepatocellular carcinoma (30). In a phase I trial of using immunotherapy KIF20A-derived peptide vaccination combined with gemcitabine revealed that KIF20A peptide-vaccine induced T-cell responses at high rates, and patients achieved stable disease with no severe adverse events (31). This implies the potential efficacy of targeting mitotic-related oncogenes in PAAD for better prognosis.
The CCNB2 is a member of the cyclin family that is required for cell cycle regulation in the G2/M phase of mitosis. Expression analysis showed significant upregulation of CCNB2 in PAAD compared to normal tissue, in addition to its association with poor prognosis in PAAD patients with high levels of expression. An in vitro study assessed the possible effect of the depletion of CCNB2 on tumor cells’ proliferation, which revealed suppression of cellular migration and growth, thus emphasizing its role in promoting cellular migration (32). The overexpression of CCNB2 along with the Aurora kinase A gene has also been shown to cause atypical mitosis, causing TP53 somatic mutations in adrenocortical carcinoma (33). Promoter hypomethylation of this gene was observed to cause higher expression in tumor tissue of hepatocellular carcinoma, and positively correlated with immunosuppressive cells infiltration, marking it as a putative prognostic marker and introducing the combination of immunotherapy and inhibitors of this gene to better prognosis (34).
The NCAPH gene is a member of the Barr gene family which acts as a regulatory subunit of the condensin complex that is required for chromosomal condensation. This gene has been associated with increased cell proliferation and inhibition of apoptosis in bladder cancer, by acting on the MEK/ERK signaling pathway (35). In this study, our findings revealed an overexpression of the NCAPH gene in PAAD, and a prognostic correlation with its level of expression, in which people with high levels of expression presented with a poorer prognosis than people with low levels of expression. In addition, the PPI analysis also showed significant enrichments in biological processes associated with chromosomal condensation. The knockdown of NCAPH in the pancreatic cell in vitro caused G2/M phase arrest and increased apoptosis, whereas another gene from this family (NCAPG) arrested hepatocellular carcinoma cells in the S phase. All the identified genes were significantly associated with mitotic regulation, suggesting a potential mechanism of PAAD pathogenesis.
All the ML classification models performed with great accuracy and high precision estimates in predicting the OS in PAAD patients based on mRNA expression of the identified genes, which provides a reliable prognostic tool for PAAD and imply the replicability and reproducibility of this model in PAAD. Instead of using one decision tree, the RFC model includes multiple decision trees and uses averaging to avoid overfitting and improve accurate predictions. All models underwent robust and unbiased multiple evaluation approaches, to validate their application in clinical practice for an early diagnosis of PAAD. Each model was cross-validated with 10-fold iterations, this method prevents model overfitting, and tests how it can be accurately used in practice and on independent datasets, by training on multiple train-test sets. All models showed narrow confidence interval accuracies implying a high precision of our results and high confidence in reproducibility for clinical practice.
Our models were based on novel genetic biomarkers, that have shown significant association with cell cycle regulation and survival in PAAD and overexpression in pancreatic cancer tissue. The classification models were trained based on the expression scores of each gene as feature variables using multiple decision trees to accurately predict the prognostic status of PAAD samples across multiple random subsets of the data that it has not been trained on. Hence, our results present a rigorous linkage between novel genomic expression and a predictive model to improve the prognostic value in PAAD.
In this study, we provided reliable findings in the favor of several strengths. First, we identified four genes that were significantly upregulated in PAAD and significantly associated with PAAD survival, and cell cycle regulation. Thus, these findings can facilitate the development of targeted therapy providing a better prognosis for PAAD. Second, we designed a predictive ML model using the RFC ensemble which is based on multiple decision trees, this presents with more accurate and stable performance and prevents overfitting. Third, we evaluated each model using robust evaluation metrics such as 10-fold cross-validation which tests the model on different subsets of the data in each iteration, and this significantly reduces bias and variance (36). The model’s accuracy was also estimated using 95% CI, which reflects the precision of our model and its reproducibility in practice. Despite the strong points in our study, these findings should be interpreted with caution in the context of several limitations. First, the PAAD dataset from TCGA contained a low number of samples with gene expression, and this would affect the model’s performance resulting in lower accuracy than in larger samples. Second, we could not train the model on regression ensembles as it requires a very large amount of data to provide good accuracy and reliable results. Finally, limited data availability of clinical variables and treatment modalities of each patient did not allow training the models on these variables which may influence the predictions.
Conclusions
In essence, we conducted a bioinformatics analysis to identify cell cycle hub genes and further validated their expression in PAAD tissue from the TCGA database. These genes included TPX2, CCNB2, KIF20A, and NCAPH, which were significantly upregulated in PAAD, and associated with poor prognosis. We also introduced and comprehensively evaluated a prognostic ML model that predicted PAAD patients’ survival based on the expression of these genes which performed with good accuracy. Our study is the first to identify genetic biomarkers associated with cell-cycle regulation in PAAD and integrate them with ML. These findings can guide the identification of these genes as prognostic signatures to aid in the development of anti-mitotic therapy targeting these hub genes.
Acknowledgments
Funding: None.
Footnote
Peer Review File: Available at https://apc.amegroups.com/article/view/10.21037/apc-22-6/prf
Conflicts of Interest: All authors have completed the ICMJE uniform disclosure form (available at https://apc.amegroups.com/article/view/10.21037/apc-22-6/coif). The authors have no conflicts of interest to declare.
Ethical Statement: The authors are accountable for all aspects of the work in ensuring that questions related to the accuracy or integrity of any part of the work are appropriately investigated and resolved. The study was conducted in accordance with the Declaration of Helsinki (as revised in 2013).
Open Access Statement: This is an Open Access article distributed in accordance with the Creative Commons Attribution-NonCommercial-NoDerivs 4.0 International License (CC BY-NC-ND 4.0), which permits the non-commercial replication and distribution of the article with the strict proviso that no changes or edits are made and the original work is properly cited (including links to both the formal publication through the relevant DOI and the license). See: https://creativecommons.org/licenses/by-nc-nd/4.0/.
References
- Siegel RL, Miller KD, Jemal A. Cancer statistics, 2020. CA Cancer J Clin 2020;70:7-30. [Crossref] [PubMed]
- Porta M, Fabregat X, Malats N, et al. Exocrine pancreatic cancer: symptoms at presentation and their relation to tumour site and stage. Clin Transl Oncol 2005;7:189-97. [Crossref] [PubMed]
- Berlin JD, Catalano P, Thomas JP, et al. Phase III study of gemcitabine in combination with fluorouracil versus gemcitabine alone in patients with advanced pancreatic carcinoma: Eastern Cooperative Oncology Group Trial E2297. J Clin Oncol 2002;20:3270-5. [Crossref] [PubMed]
- Cunningham D, Chau I, Stocken DD, et al. Phase III randomized comparison of gemcitabine versus gemcitabine plus capecitabine in patients with advanced pancreatic cancer. J Clin Oncol 2009;27:5513-8. [Crossref] [PubMed]
- Von Hoff DD, Ervin T, Arena FP, et al. Increased survival in pancreatic cancer with nab-paclitaxel plus gemcitabine. N Engl J Med 2013;369:1691-703. [Crossref] [PubMed]
- Conroy T, Desseigne F, Ychou M, et al. FOLFIRINOX versus gemcitabine for metastatic pancreatic cancer. N Engl J Med 2011;364:1817-25. [Crossref] [PubMed]
- Erkan M, Hausmann S, Michalski CW, et al. The role of stroma in pancreatic cancer: diagnostic and therapeutic implications. Nat Rev Gastroenterol Hepatol 2012;9:454-67. [Crossref] [PubMed]
- Hilmi M, Bartholin L, Neuzillet C. Immune therapies in pancreatic ductal adenocarcinoma: Where are we now? World J Gastroenterol 2018;24:2137-51. [Crossref] [PubMed]
- Ravi S, Alencar AM Jr, Arakelyan J, et al. An Update to Hallmarks of Cancer. Cureus 2022;14:e24803. [PubMed]
- Bayraktar S, Rocha Lima CM. Emerging cell-cycle inhibitors for pancreatic cancer therapy. Expert Opin Emerg Drugs 2012;17:571-82. [Crossref] [PubMed]
- Kourou K, Exarchos TP, Exarchos KP, et al. Machine learning applications in cancer prognosis and prediction. Comput Struct Biotechnol J 2015;13:8-17. [Crossref] [PubMed]
- Sun Y, Goodison S, Li J, et al. Improved breast cancer prognosis through the combination of clinical and genetic markers. Bioinformatics 2007;23:30-7. [Crossref] [PubMed]
- Simes RJ. Treatment selection for cancer patients: application of statistical decision theory to the treatment of advanced ovarian cancer. J Chronic Dis 1985;38:171-86. [Crossref] [PubMed]
- Naguib RN, Sherbet GV. Artificial neural networks in cancer research. Pathobiology 1997;65:129-39. [Crossref] [PubMed]
- Maclin PS, Dempsey J, Brooks J, et al. Using neural networks to diagnose cancer. J Med Syst 1991;15:11-9. [Crossref] [PubMed]
- Michiels S, Koscielny S, Hill C. Prediction of cancer outcome with microarrays: a multiple random validation strategy. Lancet 2005;365:488-92. [Crossref] [PubMed]
- Zhang Z, Li H, Jiang S, et al. A survey and evaluation of Web-based tools/databases for variant analysis of TCGA data. Brief Bioinform 2019;20:1524-41. [Crossref] [PubMed]
- Cerami E, Gao J, Dogrusoz U, et al. The cBio cancer genomics portal: an open platform for exploring multidimensional cancer genomics data. Cancer Discov 2012;2:401-4. [Crossref] [PubMed]
- Gao J, Aksoy BA, Dogrusoz U, et al. Integrative analysis of complex cancer genomics and clinical profiles using the cBioPortal. Sci Signal 2013;6:pl1. [Crossref] [PubMed]
- Tang Z, Kang B, Li C, et al. GEPIA2: an enhanced web server for large-scale expression profiling and interactive analysis. Nucleic Acids Res 2019;47:W556-60. [Crossref] [PubMed]
- Szklarczyk D, Gable AL, Nastou KC, et al. The STRING database in 2021: customizable protein-protein networks, and functional characterization of user-uploaded gene/measurement sets. Nucleic Acids Res 2021;49:D605-12. [Crossref] [PubMed]
- Lánczky A, Győrffy B. Web-Based Survival Analysis Tool Tailored for Medical Research (KMplot): Development and Implementation. J Med Internet Res 2021;23:e27633. [Crossref] [PubMed]
- Pedregosa F, Varoquaux G, Gramfort A, et al. Scikit-learn: Machine Learning in Python. J March Learn Res 2011;12:2825-30.
- Wachsmann MB, Pop LM, Vitetta ES. Pancreatic ductal adenocarcinoma: a review of immunologic aspects. J Investig Med 2012;60:643-63. [Crossref] [PubMed]
- Gruss OJ, Vernos I. The mechanism of spindle assembly: functions of Ran and its target TPX2. J Cell Biol 2004;166:949-55. [Crossref] [PubMed]
- Kufer TA, Nigg EA, Silljé HH. Regulation of Aurora-A kinase on the mitotic spindle. Chromosoma 2003;112:159-63. [Crossref] [PubMed]
- Warner SL, Stephens BJ, Nwokenkwo S, et al. Validation of TPX2 as a potential therapeutic target in pancreatic cancer cells. Clin Cancer Res 2009;15:6519-28. [Crossref] [PubMed]
- Liang B, Zhou Y, Jiao J, et al. Integrated Analysis of Transcriptome Data Revealed AURKA and KIF20A as Critical Genes in Medulloblastoma Progression. Front Oncol 2022;12:875521. [Crossref] [PubMed]
- Sheng Y, Wang W, Hong B, et al. Upregulation of KIF20A correlates with poor prognosis in gastric cancer. Cancer Manag Res 2018;10:6205-16. [Crossref] [PubMed]
- Kong J, Yu G, Si W, et al. Identification of a glycolysis-related gene signature for predicting prognosis in patients with hepatocellular carcinoma. BMC Cancer 2022;22:142. [Crossref] [PubMed]
- Suzuki N, Hazama S, Ueno T, et al. A phase I clinical trial of vaccination with KIF20A-derived peptide in combination with gemcitabine for patients with advanced pancreatic cancer. J Immunother 2014;37:36-42. [Crossref] [PubMed]
- Aljohani AI, Toss MS, El-Sharawy KA, et al. Upregulation of Cyclin B2 (CCNB2) in breast cancer contributes to the development of lymphovascular invasion. Am J Cancer Res 2022;12:469-89. [PubMed]
- Ikeya A, Nakashima M, Yamashita M, et al. CCNB2 and AURKA overexpression may cause atypical mitosis in Japanese cortisol-producing adrenocortical carcinoma with TP53 somatic variant. PLoS One 2020;15:e0231665. [Crossref] [PubMed]
- Zou Y, Ruan S, Jin L, et al. CDK1, CCNB1, and CCNB2 are Prognostic Biomarkers and Correlated with Immune Infiltration in Hepatocellular Carcinoma. Med Sci Monit 2020;26:e925289. [Crossref] [PubMed]
- Li B, Xiao Q, Shan L, et al. NCAPH promotes cell proliferation and inhibits cell apoptosis of bladder cancer cells through MEK/ERK signaling pathway. Cell Cycle 2022;21:427-38. [Crossref] [PubMed]
- Gupta P. Cross-Validation in Machine Learning | by Prashant Gupta. Towards Data Science. Available online: https://towardsdatascience.com/cross-validation-in-machine-learning-72924a69872f
Cite this article as: Ahmed YB, Al-Bzour AN, Qaddoura MT, Ahmed M, Alryalat SA. A prognostic machine learning model for the prediction of pancreatic adenocarcinoma prognosis based on genomic expression of four cell-cycle associated hub genes. Ann Pancreat Cancer 2023;6:4.